This is a post from multiple authors: Rebecca Fordon (The Ohio State University), Deborah Ginsberg (Harvard Law Library), Sean Harrington (University of Oklahoma), and Christine Park (Harvard Law Library)
In late 2023, several legal research databases and start-up competitors announced their versions of ChatGPT-like products, each professing that theirs would be the latest and greatest. Since then, law librarians have evaluated and tested these products ad hoc, offering meaningful anecdotal evidence of their experience, much of which can be found on this blog and others. However, one-time evaluations can be time-consuming and inconsistent across the board. Certain tools might work better for particular tasks or subject matters than others, and coming up with different test questions and tasks takes time that many librarians might not have in their daily schedules.
It is difficult to test Large-Language Models (LLMs) without back-end access to run evaluations. So to test the abilities of these products, librarians can use prompt engineering to figure out how to get desired results (controlling statutes, key cases, drafts of a memo, etc.). Some models are more successful than others at achieving specific results. However, as these models update and change, evaluations of their efficacy can change as well. Therefore, we plan to propose a typology of legal research tasks based on existing computer and information science scholarship and draft corresponding questions using the typology, with rubrics others can use to score the tools they use.
Although we ultimately plan to develop this project into an academic paper, we share here to solicit thoughts about our approach and connect with librarians who may have research problem samples to share.
Difficulty of Evaluating LLMs
Let’s break down some of the tough challenges with evaluating LLMs, particularly when it comes to their use in the legal field. First off, there’s this overarching issue of transparency—or rather, the lack thereof. We often hear about the “black box” nature of these models: you toss in your data, and a result pops out, but what happens in between remains a mystery. Open-source models allow us to leverage tools to quantify things like retrieval accuracy, text generation precision, and semantic similarity. We are unlikely to get the back-end access we need to perform these evaluations. Even if we did, the layers of advanced prompting and the combination of tools employed by vendors behind the scenes could render these evaluations essentially useless.
Even considering only the underlying models (e.g., GPT4 vs Claude), there is no standardized method to evaluate the performance of LLMs across different platforms, leading to inconsistencies. Many different leaderboards evaluate the performance of LLMs in various ways (frequently based on specific subtasks). This is kind of like trying to grade essays from unrelated classes without a rubric—what’s top-notch in one context might not cut it in another. As these technologies evolve, keeping our benchmarks up-to-date and relevant is becoming an ongoing challenge, and without uniform standards, comparing one LLM’s performance to another can feel like comparing apples to oranges.
Then there’s the psychological angle—our human biases. Paul Callister’s work sheds light on this by discussing how cognitive biases can lead us to over-rely on AI, sometimes without questioning its efficacy for our specific needs. Combine this with the output-based evaluation approach, and we’re setting ourselves up for potentially frustrating misunderstandings and errors. The bottom line is that we need some sort of framework for the average user to assess the output.
One note on methods of evaluation: just before publishing this blog post, we learned of a new study from a group of researchers at Stanford, testing the claims of legal research vendors that their retrieval-augmented generation (RAG) products are “hallucination-free.” The group created a benchmarking dataset of 202 queries, many of which were chosen for their likelihood of producing hallucinations. (For example, jurisdiction/time-specific and treatment questions were vulnerable to RAG-induced hallucinations, whereas false premise and factual recall questions were known to induce hallucinations in LLMs without RAG.) The researchers also proposed a unique way of scoring responses to measure hallucinations, as well as a typology of hallucinations. While this is an important advance in the field and provides a way to continue to test for hallucinations in legal research products, we believe hallucinations are not the only weakness in such tools. Our work aims to focus on the concrete applications of these LLMs and probe into the unique weaknesses and strengths of these tools.
The Current State of Prompt Engineering
Since the major AI products were released without a manual, we’ve all had to figure out how to use these tools from scratch. The best tool we have so far is prompt engineering. Over time, users have refined various templates to better organize questions and leverage some of the more surprising ways that AI works.
As it turns out, many of the prompt templates, tips, and tricks we use with the general commercial LLMs don’t carry over well into the legal AI sphere, at least with the commercial databases we have access to. For example, because the legal AIs we’ve tested so far won’t ask you questions, researchers may not be able to have extensive conversations with the AI (or any conversation for some of them). So that means we must devise new types of prompts that will work in the legal AI sphere, and possibly work only in the AI sphere.
We should be able to easily design effective prompts because the data set the AIs use is limited. But it’s not always clear exactly what sources the AI is using. Some databases may list how many cases they have for a certain court by year; others may say “selected cases before 1980” without explaining how they were selected. And even when the databases provide coverage, it may not be clear exactly which of those materials the AI can access.
We still need to determine what prompt templates will be most effective across legal databases. More testing is needed. However, we are limited to the specific databases we can access. While most (all?) academic law librarians have access to Lexis+ AI, Westlaw has yet to release its research product to academics.
Developing a Task Typology
Many of us may have the intuition that there are some legal research tasks for which generative AI tools are more helpful than others. For example, we may find that generative AI is great for getting a working sense of a topic, but not as great for synthesizing a rule from multiple sources. But if we wanted to test that intuition and measure how well AI performed on different tasks, we would need to first define those tasks. This is similar, by the way, to how the LegalBench project approached benchmarking legal analysis—they atomized the IRAC process for legal analysis down to component tasks that they could then measure.
After looking at the legal research literature (in particular Paul Callister’s “problem typing” schemata and AALL’s Principles and Standards for Legal Research Competency), we are beginning to assemble a list of tasks for which legal researchers might use generative AI. We will then group these tasks according to where they fall in an information retrieval schemata for search, following Marchionini (2006) & White (2024), into Find tasks (which require a simple lookup), Learn & Investigate tasks (which require sifting through results, determining relevance, and following threads), and Create, Synthesize, and Summarize tasks (a new type of task for which generative AI is well-suited).
Notably, a single legal research project may contain multiple tasks. Here are a few sample projects applying a preliminary typology:
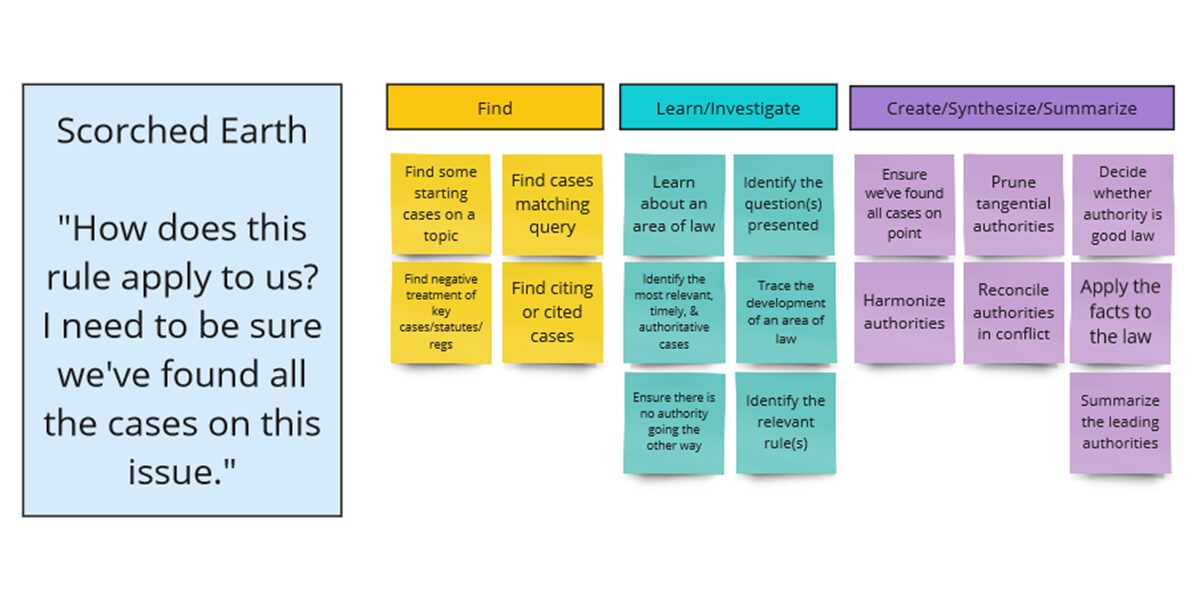
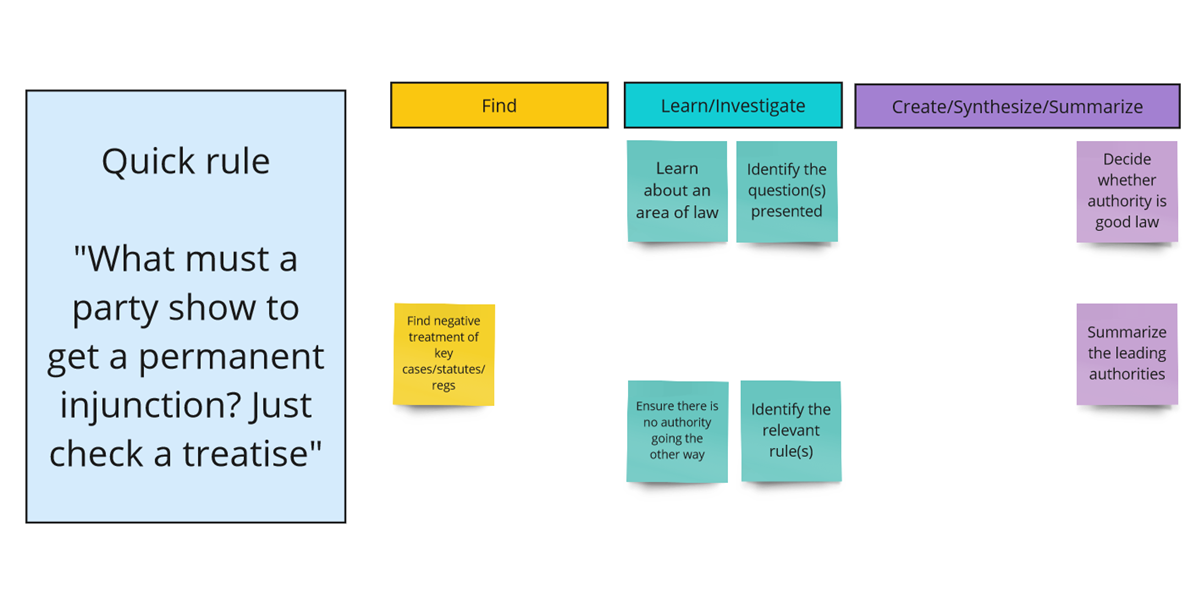
Again, we may have an initial intuition that generative AI legal research platforms, as they exist today, are not particularly helpful for some of these subtasks. For example, Lexis+AI currently cannot retrieve (let alone analyze) all citing references to a particular case. Nor could we necessarily be certain from, say, CoCounsel’s output, that it contained all cases on point. Part of the problem is that we cannot tell which tasks the platforms are performing, or the data that they have included or excluded in generating their responses. By breaking down problems into their component tasks, and assessing competency on both the whole problem and the tasks, we hope to test our intuitions.
Future Research
We plan on continually testing these LLMs using the framework we develop to identify which tasks are suitable for AIs and which are not. Additionally, we will draft questions and provide rubrics for others to use, so that they can grade AI tools. We believe that other legal AI users will find value in this framework and rubric.