Within the rapidly advancing realm of generative AI, ChatGPT is expanding its inventory of human senses available for its GPT-4 subscribers. For a mere $20 a month, you can experience its new ability to see, speak, and hear! One of these senses, the “vision” enhancement, allows the user to upload images which ChatGPT can analyize and answer questions about. Currently, YouTube is abuzz with endless creative demonstrations of this technology – solving math problems, calculating food calories in a shopping cart, identifying landmarks in photos, reading CT scans, turning sketches into art, creating fashion designs, tracking wildlife, aging or de-aging faces, detecting plant diseases, restoring old photos, suggesting interior design changes, and analyzing aerial images for urban development and natural disasters. Multiple videos are highlighting an intriguing paper, “The Dawn of LMMs: Preliminary Explorations with GPT-4V(ision),” which explores over 100 potential applications of this feature, examining how well the system performs for each.
If you don’t subscribe to ChatGPT-4, you can still check out the image analysis concept using the free open source LLaVA: Large Language Model Vision Assistant.
Originally for this blog post, I was going to examine a few examples of possible uses of this technology for legal practice. However, I got bogged down by my first example, analyzing evidence in photographs, because ChatGPT had so much to say about the image I uploaded! So, I’m focusing solely on that one.
Photograph Analysis
Photographs obviously play a huge role in legal practice. Lawyers, prosecutors, jurors, and others often rely heavily on photographs to understand events and assign blame – be it a photograph from a car accident, a crime scene, or even the positioning of a car in a parking violation scenario. Of course, in crime dramas and true crime documentaries, they either have a brilliant detective who identifies the key piece of evidence in the picture, or it is crowdsourced by the public when it appears in the news. Now, with ChatGPT’s newfound ability to “see,” it’s essentially doing its own form of crowdsourcing. Using the wisdom of countless human eyes contained in its massive training data, ChatGPT-4 can assist and provide insights that an individual’s human eyes can miss.
Example – Analysis of Office Shelf Photograph
To test its abilities, I snapped a goofy photo of a shelf in my office and quizzed ChatGPT about the things it spotted. I was pretty impressed by its descriptive abilities and knowledge about some of the items. Exploring its possible uses for like, the police, I pretended the picture was taken in the home of an international assassin, and then asked for some clues about the assassin. I think that it had some interesting ideas!
I uploaded the image and asked away!
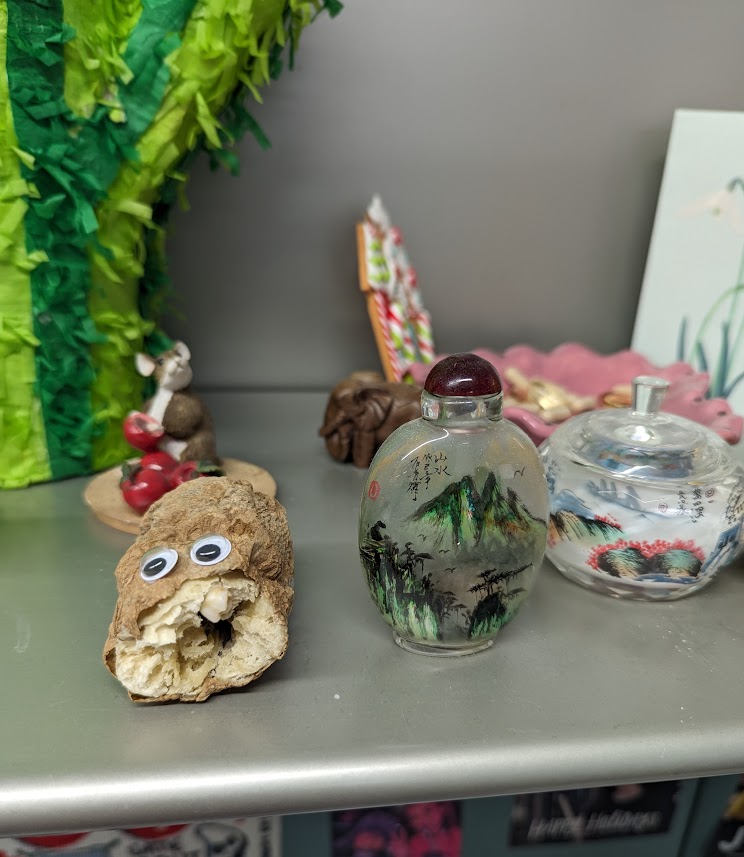
Me: Hi! Please list the items you recognize in this picture.
ChatGPT: Sure! Here are the items I recognize in the picture:
- A green piñata (or decorative item) made of fringed paper.
- A figurine of an animal, possibly a kangaroo or wallaby, holding red objects (perhaps apples).
- A piece of wood or nut with googly eyes attached, resembling a face with an open mouth.
- Two glass or ceramic bottles with decorative paintings or inscriptions.
- A candy cane or similarly striped stick.
- A wooden hand sculpture.
- A tray or plate with a pink border.
- Various stickers or decals on a gray surface, including ones that say “Night Owl”, “Juicy”, and others.
Let me know if you need more information on any of these items!
Me: Great! You see the little bottle with the nature scene on it? Tell me what you know about that picture.
ChatGPT: Certainly! The bottle you’re referring to appears to be a type of “snuff bottle.” Snuff bottles are small bottles which were primarily used in ancient China to hold powdered tobacco (snuff). These bottles became popular during the Qing Dynasty. The use of snuff was a fashion among the Chinese during this period, and they liked to carry it in small bottles like the one in your picture…. (Note: It continued for 3 more paragraphs.)
Me: Can you read the Chinese characters on the bottle?
ChatGPT: (Note: No, too small, but could read it if I uploaded another picture.)
Me: If I told you that the brown thing with eyes is a dessicated potato, could you take a guess at how long it has been drying out?
ChatGPT: While I can provide a general overview based on the image and your description, it’s important to note that accurately determining the exact duration of desiccation for a potato based solely on a photograph is challenging.
That said, when a potato dries out, it loses its moisture and shrinks, and the skin can become wrinkled and tough. Over time, as the potato continues to dry, it may become lighter in weight and more shriveled in appearance. The potato in the image does appear quite shriveled and dried out.
However, the exact duration of desiccation can vary based on several factors, including.. (Note: It goes on with a list of factors.)
Me: Yeah, that was a good guess! I accidentally left it in my office when I went to the AALL conference in July, and it is October 18 now.
ChatGPT: Ah, that makes sense! Leaving a potato in an environment like an office for several months would certainly lead to the kind of desiccation observed in the image. The absence of moisture and a relatively stable environment would have contributed to
its drying out. It’s interesting to see how everyday items can transform over time when left in certain conditions. I hope your AALL conference went well, and thanks for sharing this intriguing observation with me!
Me: Yeah, I was surprised at how it looked after a week! Can you tell what that is in the potato’s mouth?
ChatGPT: (No, didn’t guess correctly.)
Me: What if I gave you a hint? The tooth fairy.
ChatGPT: Ah, with that hint in mind, it seems the object in the potato’s mouth could represent a “tooth”! Perhaps it’s a playful representation of the potato losing a tooth and waiting for the tooth fairy. In that context, the object could be made of a variety of materials resembling a tooth, such as a piece of white foam, plastic, or even a small piece of chalk or stone. It’s a fun and creative idea!
Me: Actually, one day I was looking at the potato, and I saw one of my daughter’s baby teeth on the shelf! I don’t know when I put it there, but I do remember finding one in my wallet and thinking that I should hold on to it. So, I assume I stuck it on my shelf for safekeeping.
ChatGPT: That’s both surprising and heartwarming! Finding personal mementos in unexpected places can bring back a rush of memories and emotions. Baby teeth are often cherished keepsakes for many parents, reminding them of the fleeting nature of childhood. Your discovery adds a whimsical twist to the tradition of the tooth fairy! The potato unintentionally became a guardian for your daughter’s baby tooth. It’s a unique and memorable story you can share with her in the future.
Me: If this picture would taken by the police at the home of, say, an international assassin, would it give us any clues about the assassin?
GhatGPT: While it’s fun to imagine scenarios, it’s essential to approach such questions with a good dose of caution and skepticism. Using the image you provided, let’s hypothesize in a fictional context:
If the image were taken at the home of an “international assassin” in a crime novel or movie, investigators might look for clues in the following ways:
- Personal Touches: The dessicated potato with a baby tooth could suggest a sentimental side or a connection to family. The tooth might indicate the assassin has a child or keepsakes from their past.
- Cultural Clues: The snuff bottle with Chinese characters could hint at a connection to China, either personally, professionally, or as a destination of interest.
- Hobbies or Interests: The various trinkets and decorations might suggest that the assassin has an interest in art, culture, or collecting unique items.
- Stickers and Decals: The stickers on the gray surface might provide clues about places they’ve visited, brands they like, or organizations they support or are involved in.
- Behavioral Clues: The fact that the potato was left to desiccate for months might indicate a person who travels frequently or isn’t often at home.
- Potential Disguises: Items like the piñata could be tools for blending in at parties or social events.
However, it’s essential to note that these are purely speculative interpretations based on a fictional scenario. In a real-world context, such deductions would be highly speculative and could lead to false conclusions. Personal items in someone’s home often reflect their tastes, memories, and everyday life rather than their profession, especially one as clandestine as an “international assassin.”
Me: Thanks for your help today!
Generative AI in the Legal Sphere
Well, that was definitely interesting! Then, naturally, I turned to ChatGPT to explore potential applications in the legal sector, and it had endless ideas. Here are but a few:
Generative AI, especially with its visual capabilities, offers a plethora of applications in the legal sector:
- Document Review: Beyond just text, AI can now scan and interpret visual elements in legal documents, helping in tasks like verifying signatures or checking seals.
- Evidence Analysis: AI can be used to enhance low-quality surveillance footage, identify faces in crowded scenes, or even analyze patterns in digital data visualizations.
- Contract Visualization: Instead of lengthy text, visualize contract terms as diagrams or flowcharts, making them more digestible for clients.
- Property Assessment: Using satellite imagery, AI can assess property boundaries, land usage, or even environmental compliance.
- Accident Reconstructions: AI could recreate accident scenes in 3D based on photos and other evidence, aiding in personal injury or insurance cases.
- Copyright Compliance: Scan vast repositories of images to identify potential copyright violations or unauthorized use of intellectual property.
- Client Interactions: Use AI to generate visual explanations of complex legal scenarios, helping clients grasp intricate details through interactive diagrams or simulations.
- Forensic Art: Create composites or age-progression photos in missing person cases or help juries visualize crime scenes.
- Legal Research: AI can scan through visually rich documents like patents, identifying key diagrams or illustrations that could be crucial in patent litigation.”
Conclusion
With tools like ChatGPT expanding their capabilities, the intersection of AI and law is clear. ChatGPT’s new features highlight potential efficiencies and improvements for legal processes!